New Method for Testing Predictability in Financial Markets
Published on Sun Aug 06 2023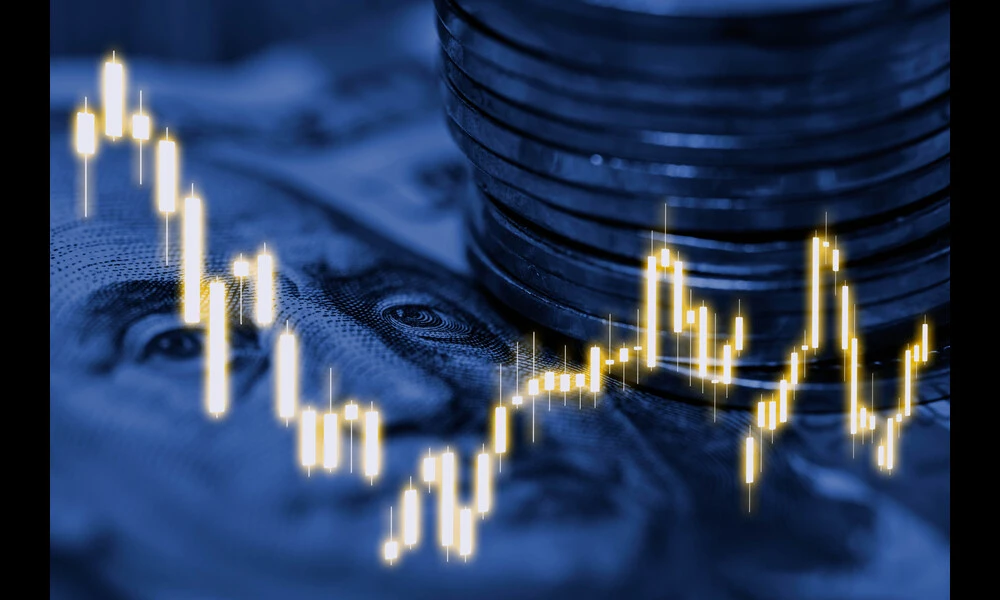
New research has developed a robust method for testing predictability in financial markets, even when there are fluctuations in the parameters being measured. The study, titled "Predictability Tests Robust against Parameter Instability," focused on the stock market predictability puzzle for the US equity premium. The researchers showed that traditional tests based on ordinary least squares (OLS) estimators can converge to a nonstandard distribution when predictors are nonstationary. However, using instrumental variable extension (IVX) estimators, the tests were able to filter out the persistence of parameters under certain restrictions.
The stock predictability puzzle is an important area of research in financial economics. Previous studies have demonstrated that the forecast performance of predictive regressions with macroeconomic variables as predictors varies depending on the business cycle. Additionally, some periods have shown episodic predictability, where predictors have no ability to predict during certain periods but then "switch on" during others. This phenomenon is linked to parameter instability in time series models.
The research aimed to investigate how the degree of persistence of predictors affects predictability testing when there is parameter instability. The authors developed a framework for jointly testing predictability and structural break using a Wald type statistic. They demonstrated that the test is robust to the persistence properties of predictors and can provide valuable insights into predictability even in the presence of parameter instability.
The findings of the research were validated through extensive Monte Carlo experiments, comparing the performance of the tests under both OLS and IVX estimators. The critical values for the tests were computed using standard bootstrap methods. The study also examined the finite-sample properties of the tests and their application to the stock market predictability puzzle.
This research contributes to the existing literature on joint predictability and parameter instability testing. It provides analytical tractable asymptotic theory for testing predictability when considering nonstationary regressors. By comparing the results with previous work in these fields, the study highlights some interesting findings not previously presented. The methods proposed in this research have the potential to improve the accuracy of predictability testing, particularly when dealing with highly persistent regressors.
Conducting inference on the regression coefficients of predictive regression models with highly persistent regressors can lead to nonstandard limiting distributions. However, the robust testing methodology proposed in this research ensures that the limiting distribution of structural break tests is free from any nuisance parameters. The study concludes by emphasizing the importance of this research for empirical finance applications, where persistent properties and parameter instability often exist in the available information about current and future economic conditions.