How Spatio-Temporal Graph Neural Networks are Transforming Smart Cities
Published on Wed Dec 06 2023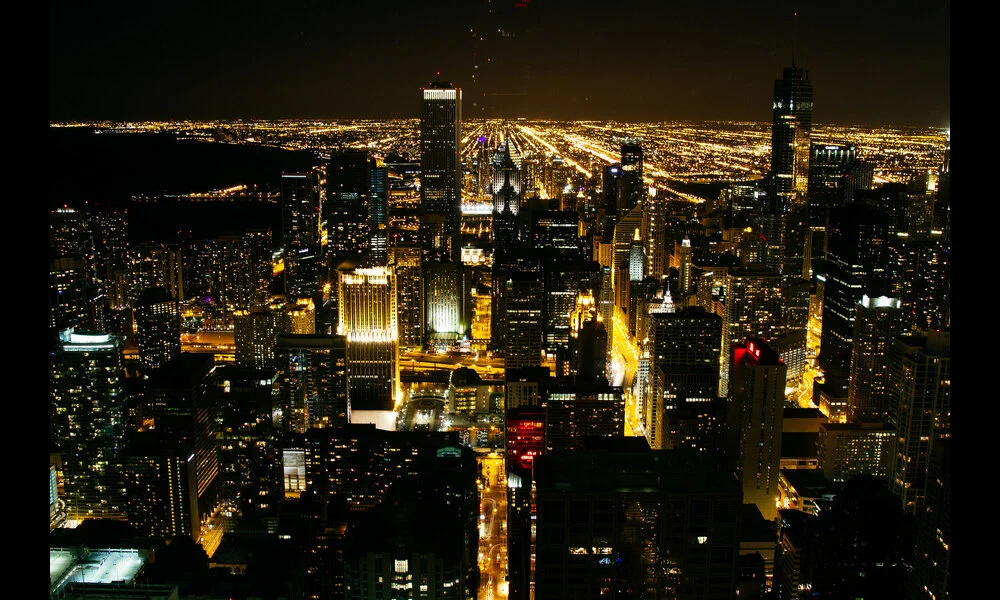
Urban planners, environmentalists, and public safety officials are often faced with the Herculean task of predicting how a city pulse like a living organism, with traffic patterns ebbing and flowing or air quality changing with the weather. Understanding and forecasting these patterns are essential for smart city initiatives aimed at improving quality of life and sustainability. A substantial leap in predictive urban computing has been achieved through a novel class of AI models known as Spatio-Temporal Graph Neural Networks (STGNN). Heralding a new dawn in intelligent urban management, a comprehensive survey of STGNNs has meticulously charted the advancements, appraised the technologies, and outlined the promising future of predictive learning in smart cities.
The essence of STGNNs lies in their unparalleled finesse in deciphering the intricate spatial and temporal threads woven into the fabric of urban data – capturing how elements interact over time and space. Unlike traditional models that stumble while processing the non-linear and non-Euclidean nature of such data, STGNNs thrive in this environment, proving to be an indispensable tool in a variety of urban applications. From smoothing traffic to predicting pollution levels or anticipating the need for emergency services, these networks are helping cities become more responsive and anticipatory to the needs of their inhabitants.
The key to STGNN's success is attributed to its graph-based approach, which mirrors the interconnectedness of urban elements – be it roads, environmental sensors, or public services. Furthermore, the integration with other advanced learning technologies boosts their accuracy and versatility. The paper details how these hybrid frameworks, including adversarial and meta-learning, supercharge STGNNs to new heights of performance and offer tantalizing glimpses into a future where digital twin cities and metaverse technologies are integrated into the urban fabric.
While STGNNs have proven to be a boon for urban computing, the survey does not shy away from identifying limitations in current research, such as the lack of interpretability, physical constraints, and scalability. Here lies the value of the findings: as the survey points out future directions, it becomes a beacon for ensuing research, signaling areas ripe for innovation. The challenges outlined in this paper, ranging from interpretability and calibration to addressing physical constraints and enhancing scalability, are not just academic concerns. They represent real-world hurdles that, when overcome, could substantially refine the predictive models that urban centers rely on to make decisions that affect millions of lives.
In summary, this survey doesn't just report on the state of STGNNs; it opens a dialogue on how to bridge the gap between the latest technological advances and the day-to-day realities of urban living. It's a call to action for researchers, city planners, and technology developers to push the boundaries of what's possible in making our cities smarter, safer, and more sustainable.