New Research Uncovers the Secret to Robust Motor Control
Published on Mon Jul 10 2023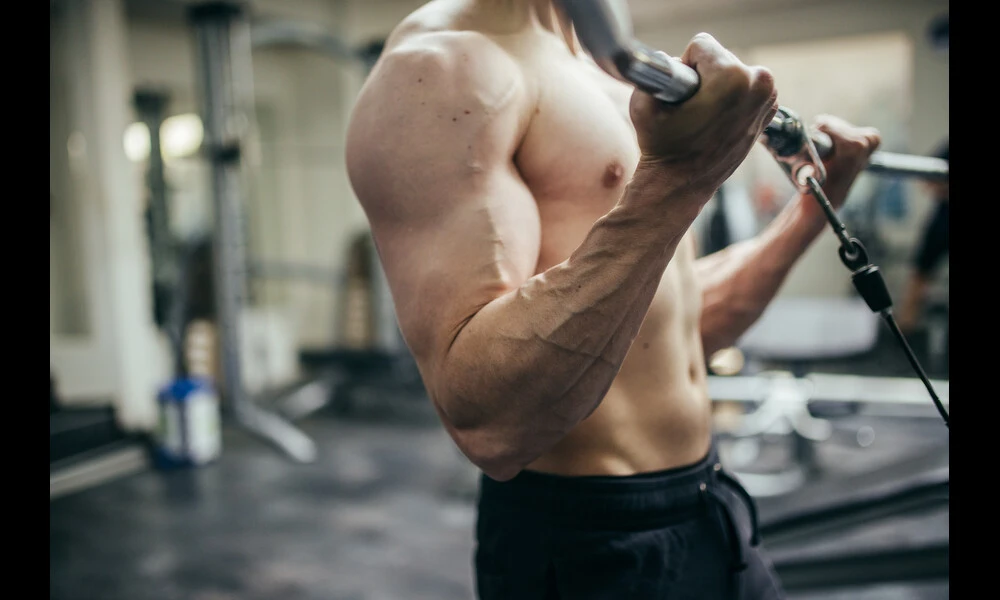
New research suggests that the secret to robust motor control lies in the coordination of muscle activity. A team of researchers from Meta AI has developed a Synergistic Action Representation (SAR) that mimics the muscle synergies found in humans and animals, and they have used it to train highly effective continuous control policies for complex tasks. The researchers trained physiologically accurate models of the human hand and legs on a range of tasks, including locomotion on diverse terrains and multi-object manipulation. The results were impressive, with the SAR-exploiting policies consistently outperforming traditional end-to-end reinforcement learning methods.
In locomotion tasks, the SAR-enabled policies achieved robust movement on various terrains, such as stairs and hills, with an unprecedented level of efficiency. Similarly, when it came to manipulating multiple objects, the SAR-exploiting policies significantly outperformed baseline approaches. What's more, these policies were able to generalize to out-of-domain environmental conditions, while policies that did not adopt SAR failed to do so. In fact, the SAR method proved to be so effective that it even extended beyond physiological control and delivered remarkable performance in robotic manipulation and full-body humanoid locomotion tasks.
This groundbreaking research not only sheds light on how humans and animals have evolved to achieve such impressive motor control but also provides a promising approach for developing generalist embodied agents. By leveraging the concept of muscle synergies, these agents can learn highly complex behaviors with improved sample efficiency. The potential applications of this discovery are vast, ranging from robotics to AI-controlled systems. The method developed by the researchers can be applied to a diverse range of musculoskeletal tasks, and the concept of muscle synergies as a form of transfer learning opens up exciting avenues for future research.