AI Speeds Up Screening Process in Systematic Reviews by 70%
Published on Thu Aug 24 2023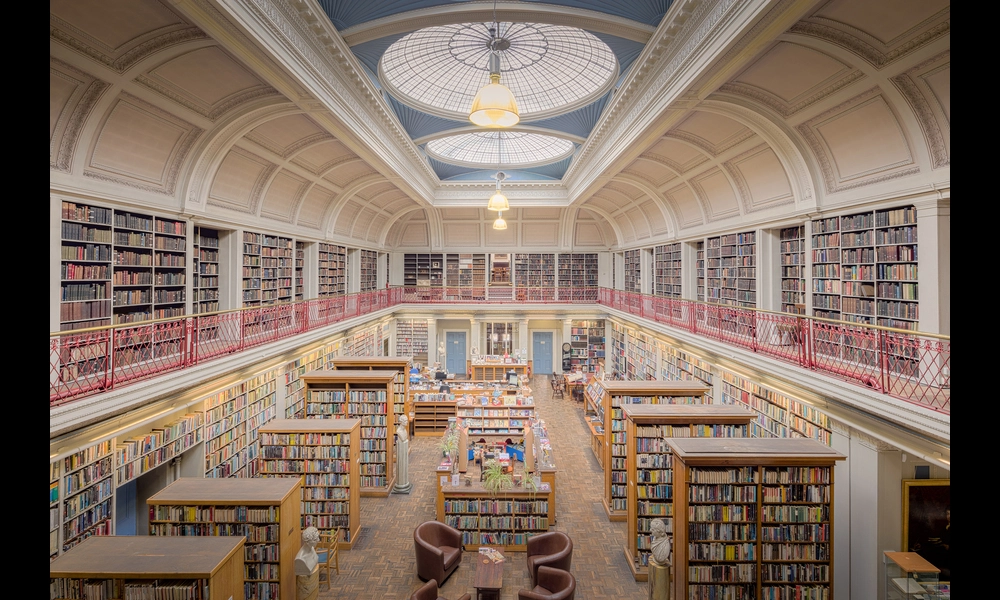
Researchers have developed a new artificial intelligence (AI) software called Sciome SWIFT-ActiveScreener (ActiveScreener) that can potentially speed up the screening process in systematic reviews (SRs) while maintaining accuracy and adherence to standardized protocols. SRs involve synthesizing empirical evidence to answer specific research questions, but they can be time-consuming and require extensive resources. ActiveScreener uses machine learning to predict whether articles should be included or excluded in a SR, reducing screening time and effort by up to 70%. In a recent evaluation of its accuracy and usability in a large-scale SR of mental health outcomes following treatment for PTSD, ActiveScreener exceeded the expected 95% accuracy and was praised as user-friendly by both inexperienced and experienced screeners. These findings suggest that ActiveScreener may save significant time and human resources in the screening phase of SRs.
During the screening process of a SR, researchers review thousands of articles against strict inclusion and exclusion criteria to determine eligibility for inclusion. AI software like ActiveScreener aim to expedite this process. While ActiveScreener does not eliminate human involvement, it significantly reduces time and resources by using a machine learning algorithm to prioritize articles for screening. By analyzing patterns of screening, ActiveScreener builds a predictive model, estimating articles for inclusion or exclusion. Past research has shown its effectiveness in physical health literature reviews.
This particular study aimed to evaluate the precision and usability of ActiveScreener in a mental health treatment SR. The results revealed that ActiveScreener performed above its expected accuracy, reaching an accuracy of 97.9% in predicting inclusion or exclusion. Discrepancies between the machine learning algorithm and human screeners were minimal. The usability of ActiveScreener was also positively evaluated, with screeners finding it easy to learn and use. However, software glitches were reported, and additional features, such as bulk upload and templates for data extraction, were suggested to further reduce human resource requirements.
While ActiveScreener shows promise in accelerating the screening phase of SRs, researchers must ensure the clarity of inclusion and exclusion criteria during training and screening stages to maximize accuracy. Additionally, the deduplication function of ActiveScreener may require further development to cover punctuation, which can result in duplicate references when screening. It is important to note that ActiveScreener's time and resource savings currently only apply to the initial screening phase of SRs at the title and abstract stage. Nevertheless, ActiveScreener presents itself as a reliable and user-friendly platform that can save considerable time and resources when used appropriately in SRs.