New Research Shows Promise in Automated Deception Detection
Published on Sun Sep 03 2023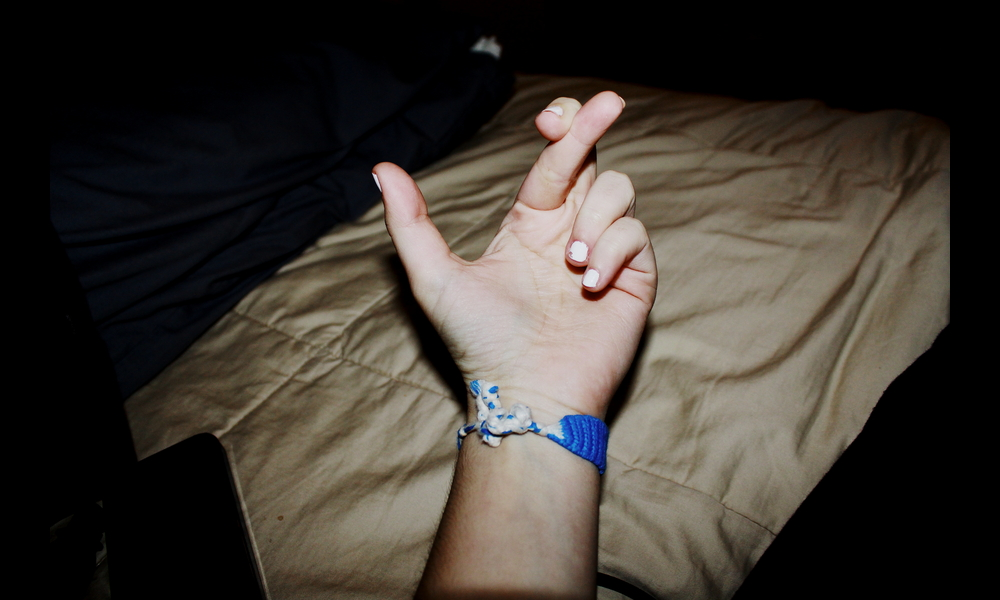
Detecting deception has long been an important issue in many areas of our lives, from courtrooms to job interviews. Traditional methods of deception detection, such as analyzing physical cues and using polygraph machines, have their limitations. However, a new preprint paper titled "Voting-based Multimodal Automatic Deception Detection" proposes a promising solution using machine learning and deep learning techniques.
The researchers behind the paper developed a voting-based method for automatic deception detection from videos using audio, visual, and lexical features. They split video samples into frames of images, audio, and manuscripts, and built three models for deception detection: a Convolutional Neural Network (CNN) for images, a Support Vector Machine (SVM) on Mel spectrograms for audio, and a Word2Vec on SVM for text.
The results of the experiments were impressive. The proposed solution outperformed previous state-of-the-art models in detecting deception. On the Real-life Trial Dataset, the accuracy achieved for images, audio, and text were 97%, 96%, and 92% respectively. On the Miami University Deception Detection Dataset, the accuracy for videos, audio, and text were 97%, 82%, and 73% respectively.
By combining the results from the three models using a fusion equation, the overall accuracy reached around 90% on the Real-life Trial Dataset and 77% on the Miami University Deception Detection Dataset. These results suggest that automated deception detection using multimodal features and machine learning algorithms can provide a practical and accurate solution for detecting lies in various situations.
The implications of this research are significant. Automated deception detection can help prevent wrongful accusations and improve the accuracy of hiring decisions. The proposed method has the potential to be used in courtrooms, job interviews, and other scenarios where detecting deception is crucial.
This study is part of a growing body of research aiming to automate the process of deception detection. Previous studies have explored markers of deception in human-robot interactions and clues in verbal, vocal, and facial cues. By combining these findings with advanced machine learning techniques, researchers are making strides in developing more reliable and practical methods for detecting lies.
While automated deception detection is still in the research stage, this latest paper brings us one step closer to a future where machines can accurately detect deception. With further development and refinement, this technology has the potential to revolutionize the way we identify truth from falsehoods in various aspects of our lives.