New Dataset Could Help Detect Damage in Ropes
Published on Thu Oct 05 2023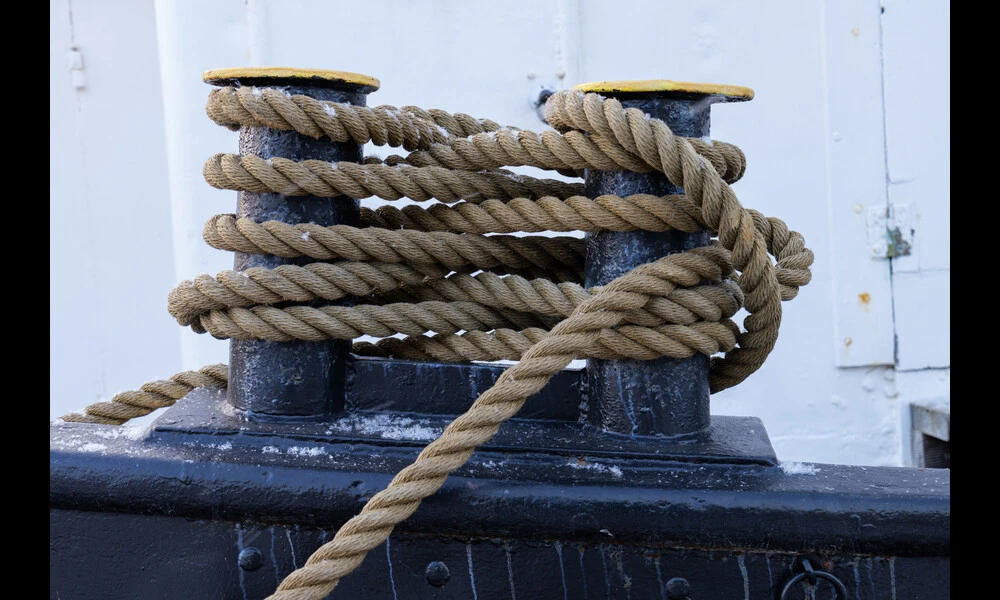
A newly generated dataset of nearly 7,000 images, representing both normal and defective synthetic fibre ropes (SFRs), is set to revolutionize the way these ropes are inspected for potential safety risks. SFRs are widely used in industries such as offshore and wind turbine, where their structural integrity is of paramount importance. The presence of defects in these ropes can compromise their strength and pose significant safety risks. However, due to their size and weight, detaching and inspecting them frequently is often impractical. This dataset aims to address this challenge by providing a resource to support computer vision applications for defect detection in SFRs.
The dataset includes a wide array of defect scenarios that can occur throughout the operational lifespan of SFRs. It encompasses various types of defects, such as placking, cut strands, chafing, compressions, core outs, and normal. By utilizing this dataset, researchers and practitioners can develop and evaluate algorithms that accurately identify and classify these defects. This will enable more efficient and reliable defect detection methods, leading to improved safety and risk mitigation in industries that rely on SFRs.
The value of this dataset lies in its ability to facilitate the development of automated defect detection systems that outperform traditional visual inspection methods. It serves as a benchmark for evaluating the performance of different defect detection techniques and promotes collaboration and knowledge sharing among industry experts, researchers, and stakeholders involved in SFR applications. Additionally, the availability of this dataset may encourage the adoption of standardized testing protocols and best practices for the inspection of SFRs across industries.
The dataset, consisting of 6,942 labeled images, can be utilized for a range of computer vision applications, including object detection, classification, and segmentation. By training and testing algorithms using this dataset, researchers can ensure accurate and efficient defect detection tailored to the unique challenges of inspecting large, heavy ropes. Ultimately, this dataset aims to improve the safety and efficiency of utilizing SFRs in various applications, ensuring their optimal condition and preventing potential failures.
The data was collected using a specialized camera setup, capturing images at different motor speeds to simulate various operating conditions that SFRs may experience. Ten different SFRs, each with a length of 8 meters, were used to generate the dataset, ensuring a comprehensive representation of different defect classes. The dataset has been compiled into separate folders, categorized by defect type or severity level, and is publicly accessible for researchers and practitioners to utilize for their studies.
With this innovative dataset, the field of SFR inspection and condition monitoring is poised for advancements in automated defect detection methods. By harnessing the power of computer vision, these methods will enhance safety, improve efficiency, and pave the way for the widespread adoption of standardized testing protocols in industries that rely on SFRs.