Enhancing AI Safety: New Technique Improves Detection of Unfamiliar Data in Neural Networks
Published on Thu Jan 11 2024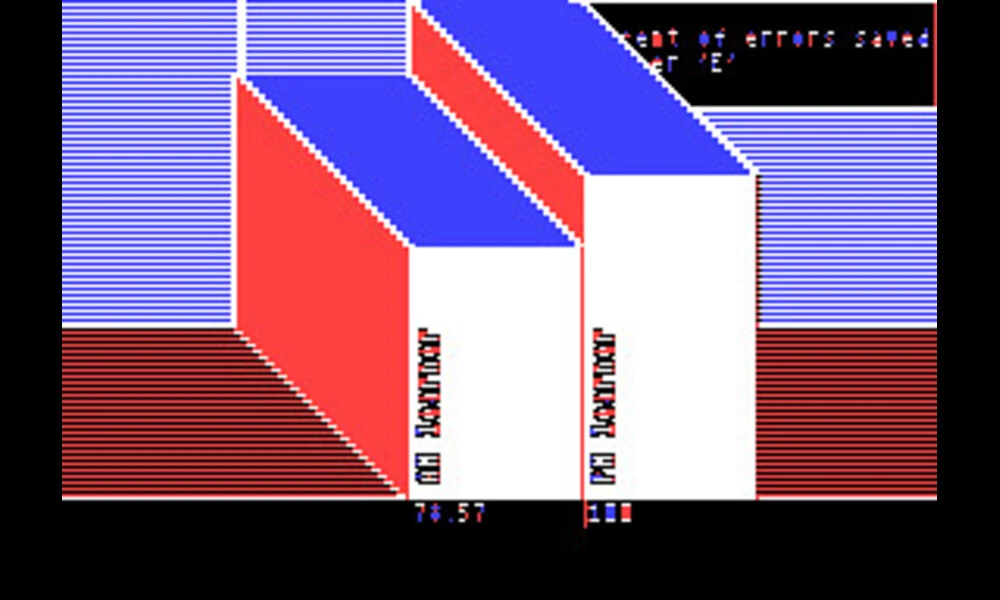
In the quest to safeguard artificial intelligence from unexpected events, researchers at the University of Toronto, Vector Institute, and Borealis AI have taken a significant stride forward. They've tapped into the essence of what indicates normal operation, developing a new approach to identify when AI systems encounter something they haven't seen before, known as out-of-distribution (OOD) data. OOD detection is crucial for real-world applications of AI, where encountering the unknown can lead to failures or unsafe decisions.
Deep generative models (DGMs), which are often used for such tasks, can falter by mistakenly identifying OOD data as familiar. But this team didn't resign to the status quo. Instead, they introduced the "likelihood path (LPath) principle", a revolutionary concept that refines the well-known statistical likelihood principle. The LPath principle suggests that by focusing on the journey, or path, that data takes through a neural network — rather than just the endpoint—we can extract more meaningful insights that signal when data is out of the ordinary.
Their investigation, using a case study on variational autoencoders (VAEs), reveals that the success doesn't solely rest in the intricate design or size of the models, but rather in the statistical nuances within the model's operations. They've crafted theoretical tools like "nearly essential support" and "essential distance" which provide a mathematical guarantee for their method's ability to spotlight OOD data, even when the density estimates are less than perfect. This research could be the key to unlocking safer, more reliable, and theoretically sound AI applications across a variety of fields.
To corroborate their findings, the researchers put their techniques through rigorous testing, and the results were impressive. Their seemingly simple method delivered superior performances against the current state-of-the-art, especially in scenarios where traditional VAEs would typically stumble. This achievement suggests promising advancements in AI safety and reliability, opening the door to AI systems that better understand their limits and, crucially, when they might be stepping beyond them.
In an era where AI's reach extends into ever more critical aspects of life, the team's work provides a scientifically grounded beacon, lighting the path towards trustworthiness in artificial intelligence. The full implications of their discovery are still unraveling, but the researchers are hopeful that they have laid the groundwork for future innovations in OOD detection.