Right to Be Forgotten Impacts On Fairness in AI Systems
Published on Mon Feb 05 2024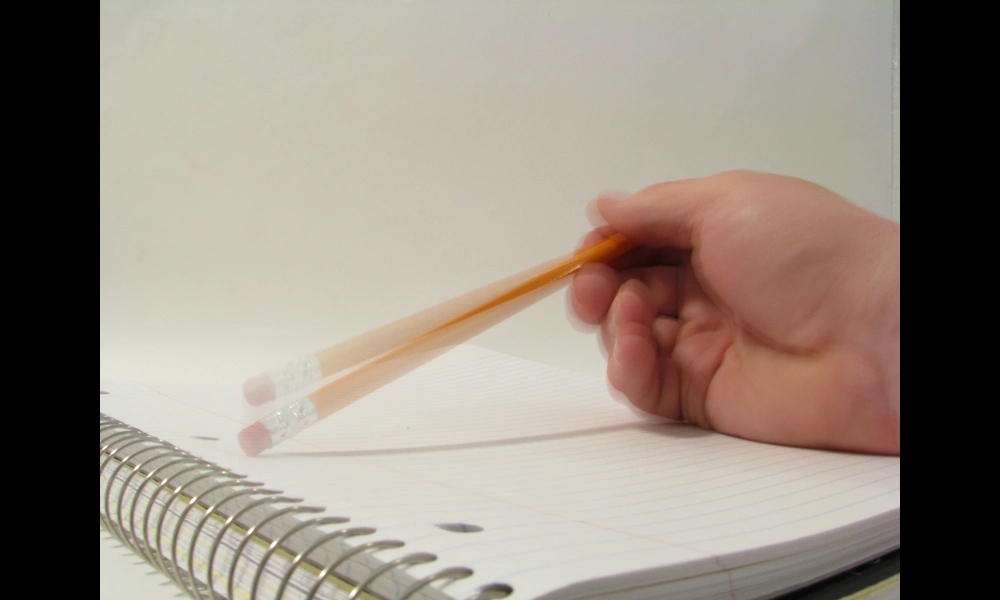
In a world increasingly governed by digital data, privacy and fairness have become paramount concerns in the tech industry, particularly with the advent of machine learning systems. Recognizing the critical need for these systems to "forget" data upon request—a concept known as the "right to be forgotten" or RTBF—researchers at CSIRO’s Data61 have delved into the fairness implications of machine unlearning methods. Their groundbreaking study, titled "To Be Forgotten or To Be Fair: Unveiling Fairness Implications of Machine Unlearning Methods," marks the first of its kind to explore how machine unlearning affects fairness within artificial intelligence (AI) systems.
Machine unlearning aims to erase sensitive data from machine learning models efficiently, complying with RTBF requests. However, this process could unintentionally skew the fairness of AI systems, raising ethical concerns. The study concentrates on two machine unlearning techniques, comparing them against traditional retraining methods over various fairness datasets and deletion strategies. The findings reveal that one method, SISA, consistently leads to better fairness outcomes under non-uniform data deletions compared to others.
For the average reader, the significance of these findings cannot be overstated. As we entrust machines with increasingly critical tasks—from hiring decisions to loan approvals—the integrity of their decisions hinges not just on the accuracy but on fairness. This research illuminates the potential fairness trade-offs involved in ensuring data privacy through machine unlearning, guiding software engineers towards more informed, ethical choices in AI development.
Furthermore, these revelations underscore the complex relationship between data privacy and fairness in AI systems. They prompt a crucial dialogue on the necessity of balancing these sometimes competing interests, paving the way for future investigations to refine machine unlearning techniques for the betterment of society.
In essence, the researchers from CSIRO’s Data61 extend an invitation to the tech community: to engage with the intricate dynamics of AI ethics and contribute to evolving AI systems that honor both our right to privacy and our demand for fairness. This pioneering study not only charts a path toward more equitable AI but also challenges us to rethink the consequences of our digital footprints in the age of artificial intelligence.