Researchers Use ChatGPT For Film Recommendations
Published on Wed Apr 24 2024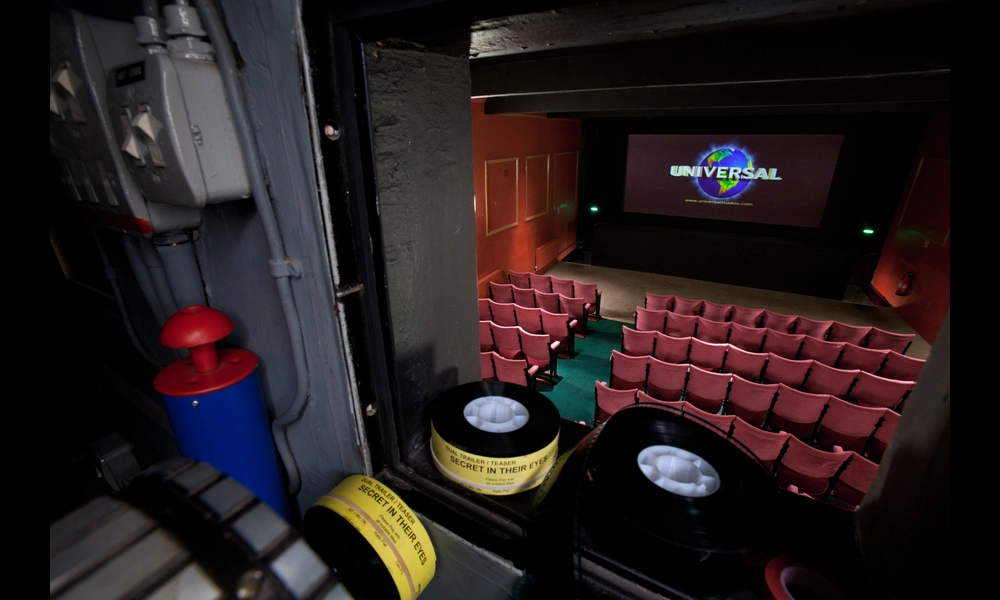
In a new study by Yashar Deldjoo from the Polytechnic University of Bari, Italy, the spotlight is turned on the biases and nuances of Recommender Systems based on ChatGPT, compared with traditional Collaborative Filtering (CF) models in the realm of movie recommendations. This research, titled "Understanding Biases in ChatGPT-based Recommender Systems: Provider Fairness, Temporal Stability, and Recency," ventures deep into the mechanics of Large Language Model (LLM)-driven recommendations, dissecting how prompt designs influence the accuracy, fairness, diversity, and temporal acuity of film suggestions.
The analysis uncovers intriguing facets of ChatGPT's recommendation tendencies, including a pronounced inclination towards promoting recent movies, especially those post-2000, alongside a propensity for genres such as 'Drama,' 'Comedy,' and 'Romance.' A striking find is that role-based prompts significantly elevate fairness and diversity in suggestions, effectively clipping the wings of popularity biases. Additionally, the study showcases how strategies like motivating reasoning or adopting Chain of Thought (COT) prompting could not only refine GPT-based recommendations but also nudge them closer to the performance benchmarks set by traditional CF models.
One of the research's revelations is that integrating explicit ratings in prompts appears to bolster the personalization quality of recommendations, offering a more nuanced reflection of individual preferences. Yet, despite these advances, GPT-based models exhibit a stronger preference for newer movie genres, a stance speculated to arise from their broad training on diverse, contemporary datasets. This characteristic underscores a potential avenue for enhancing model performance by limiting recommendations to items within a given dataset, thereby elevating both accuracy and relevance.
The study holds significant value for those navigating the complexities of recommendation systems, particularly in optimizing the use of emerging RecLLMs. It illuminates the pathways through which prompt design can substantially alter recommendation outcomes, offering a beacon for future research aiming to marry the accuracy, fairness, and user experience components in such systems. This exploration into ChatGPT's biases, quirks, and potential in movie recommendations not only enriches our understanding of LLM-based systems but also paves the way for crafting more balanced, varied, and up-to-date recommender systems.