Dynamic Safety Assurance for Autonomous Systems: A New Method Adapts to Changing Conditions
Published on Fri Jul 05 2024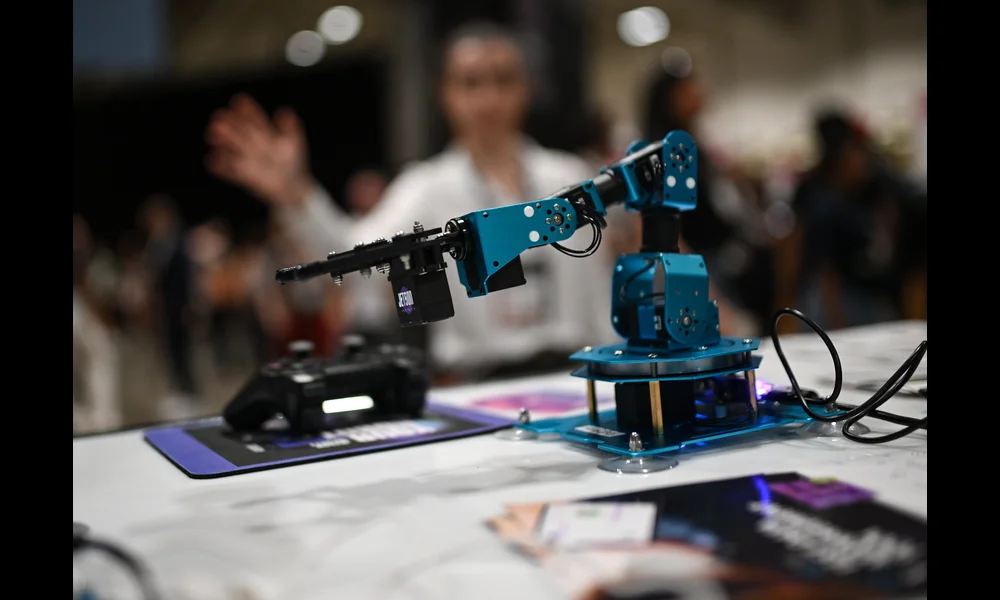
Autonomous systems, like self-driving cars and drones, undergo continuous changes in their operating environment and system parameters, making ensuring their safety a paramount but challenging task. Traditionally, safety analyses assume static conditions, a significant limitation in real-world applications where changes are inevitable. A groundbreaking study led by Javier Borquez, Kensuke Nakamura, and Somil Bansal introduces a novel approach to dynamically update safety assurances through parameter-conditioned reachable sets, revolutionizing how we think about safety in autonomous systems. Their method, meant for real-time applications, leverages advancements in reachability analysis and deep learning to adapt to changes in the system's environment or dynamics, ensuring sustained safety without starting from scratch.
Reachability analysis, a method used to ensure system safety by identifying potential unsafe states, traditionally does not account for real-time environmental or systemic changes. The research team's innovation treats variables such as environmental conditions and system dynamics as extra dimensions in the analysis, enabling the creation of a family of reachable sets conditioned on these parameters. This advance means that as conditions change, an autonomous system can immediately refer to the precomputed sets relevant to its current state, allowing for ongoing real-time safety assurance.
Implementing this system involves complex computational tasks handled offline through a deep learning framework named DeepReach, which estimates reachability problems even in high-dimensional spaces efficiently. The researchers demonstrated the effectiveness of their approach through various simulations, including scenarios involving autonomous vehicles and drones, where conditions such as wind strength or obstacles' positions change unpredictably. These applications showed that the system could dynamically adapt to new conditions, updating its safety measures to avoid potential hazards successfully.
The potential implications of parameter-conditioned reachable sets are vast. Beyond improving the safety of autonomous transportation, this method could enhance the reliability of robots in dynamic environments such as factories or warehouses, where conditions change rapidly and unpredictably. Moreover, as this approach can be applied universally to any autonomous system encountering variable conditions, it paves the way for safer integration of these technologies into our daily lives.
While the research provides a robust framework for enhancing autonomous system safety in dynamic environments, the authors note the need for further validation. Future directions include formalizing safety assurances for the derived reachable sets and expanding the method to account for environments that cannot be easily parameterized. Additionally, real-world testing on hardware systems will be crucial to transition from theory to practice. This study represents a significant step forward in ensuring the safety of autonomous systems, with promising applications across numerous domains.