Transform-Based Layers Boost CNN Efficiency and Accuracy, Applications for Computer Vision
Published on Fri Jul 05 2024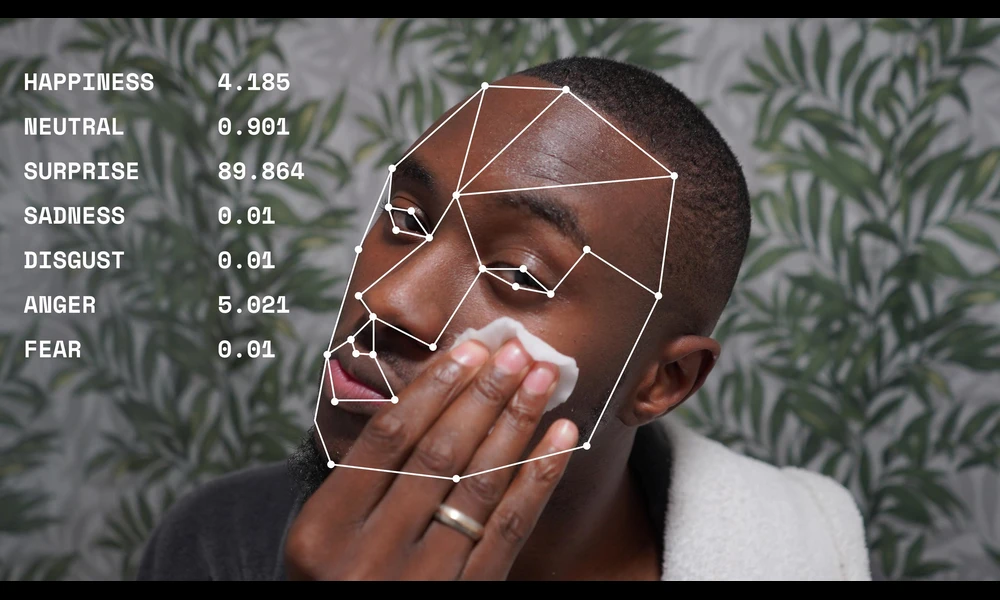
Advancements in Artificial Intelligence (AI) and machine learning have dramatically reshaped the landscape of computer vision, with Convolutional Neural Networks (CNNs) standing at the forefront of this transformation. A novel research paper introduces a groundbreaking method that could make CNNs, especially Residual Networks (ResNets), more efficient and accurate. Penned by Hongyi Pan and colleagues, the study unveils transform-based perceptual layers as a more computationally light alternative to the conventional Conv2D layers used in CNNs.
The core of this innovative approach lies in the implementation of orthogonal transforms—namely, the Discrete Cosine Transform (DST), Hadamard transform (HT), and the biorthogonal Block Wavelet Transform (BWT)—within neural network layers. By leveraging convolution theorems, these layers perform convolution filtering in the transform domain using straightforward element-wise multiplications, significantly reducing the number of parameters and multiplication operations required. Not only does this novel method bolster efficiency, but it has also shown to improve the accuracy of ResNets on benchmark image classification tasks like ImageNet-1K.
One of the standout features of these transform-based layers is their specificity to spatial locations and channels. Traditional Conv2D layers lack this kind of specificity, oftentimes leading to redundancy and inefficiency in feature extraction for different spatial contexts. The proposed model addresses this by being both location-specific and channel-specific, ensuring that its convolutional operations are more tailored and efficient.
Moreover, these layers introduced by Pan and colleagues are adaptable, proving applicable as additional components in conventional ResNets to elevate classification accuracy further with only a minor increase in parameter count. This highlights not only the efficiency and accuracy improvements but also the versatility of the proposed approach in enhancing existing deep learning models.
This breakthrough presents a promising avenue for the future of CNNs, making deep learning models more accessible by reducing computational costs without sacrificing performance. As AI continues to evolve and find applications across a vast array of fields, techniques like the one proposed by Pan and his team are crucial in pushing the boundaries of what's possible, making efficient and powerful computation more attainable.