theft: A New Package for Time-Series Analysis in R
Published on Mon Jul 10 2023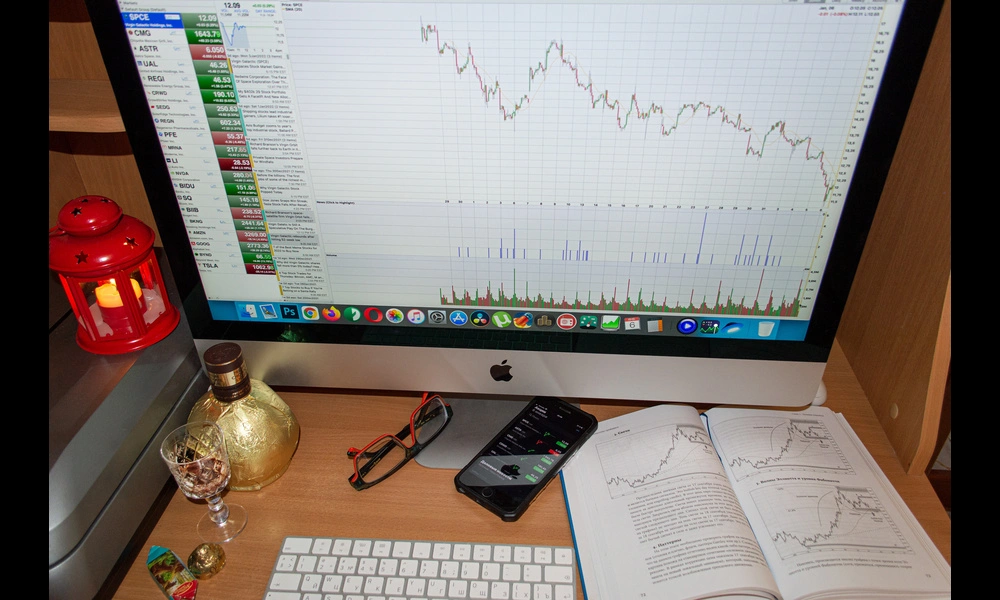
Researchers have developed a new statistical software package called theft: Tools for Handling Extraction of Features from Time series, which aims to simplify and streamline time-series analysis. Time series, or repeated measurements taken over time, are commonly used in various fields, from neuroscience to environmental studies. Analyzing time series involves calculating a set of summary statistics or 'features', which can then be used to train classification algorithms or perform other statistical learning tasks.
One of the challenges in time-series analysis is the need to use multiple software packages that often have different syntax and programming languages. This can be time-consuming and limit the accessibility of these tools. theft addresses this issue by unifying six open-source time-series feature sets, including catch22, feasts, tsfeatures, Kats, tsfresh, and TSFEL, into a single software package for the R programming language. The package also provides a comprehensive range of analyses and data visualization templates, making it easier for researchers to understand and interpret the extracted features.
By providing a standardized framework for feature-based time-series analysis, theft allows researchers to quantify and interpret the structure of time series more effectively. It eliminates the need to use multiple software libraries and streamlines the workflow for conducting feature-based analysis. The package has been demonstrated on various classification problems, showcasing its ability to extract, process, normalize, and classify time-series features. Moreover, theft offers flexibility for users to adapt the analysis templates or build new functionality for their specific research needs.
The open-source nature of theft ensures that researchers can access and contribute to the software's ongoing development. As new and more powerful features are developed, they can be incorporated into theft, expanding its capabilities for solving a wide range of problems using time-series data. The source code for theft is available on GitHub, allowing interested users to download and explore the software further.
With the increasing volume and complexity of large time-series datasets in various scientific and industrial fields, theft provides a valuable tool for researchers to comprehensively analyze and interpret the informative structure within their data. It simplifies the process of time-series feature extraction and analysis, making it more accessible to a wider range of researchers.