NA-SODINN Deep Learning Algorithm for Detecting Exoplanets
Published on Thu Oct 26 2023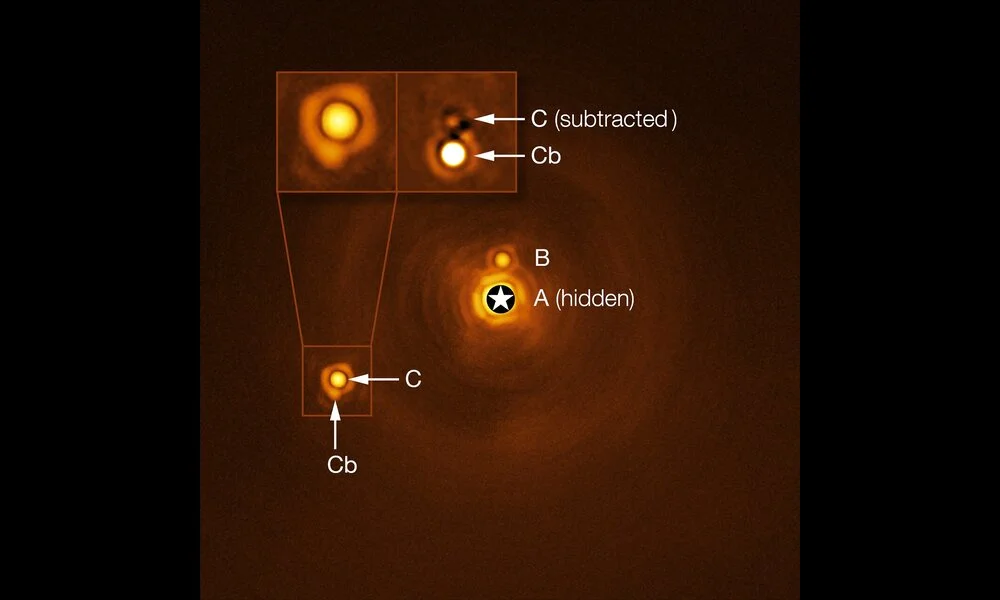
New research published in the preprint paper "NA-SODINN: A deep learning algorithm for exoplanet image detection based on residual noise regimes" explores a new approach to improving the detection of exoplanets in high-contrast images. The study, conducted by a team of astronomers, focuses on the identification and analysis of different noise regimes in processed images to enhance the performance of detection algorithms.
Currently, direct imaging of exoplanets is made possible by advanced techniques such as extreme adaptive optics and dedicated high-contrast imaging instruments. However, these images are still affected by various sources of noise, including residual stellar light, atmospheric turbulence, and optical aberrations. This noise makes it challenging to distinguish true exoplanet signals from speckles, which are scattered starlight blobs that can mimic the appearance of exoplanets.
To overcome this challenge, the researchers propose a new deep learning algorithm called NA-SODINN. This algorithm uses a convolutional neural network (CNN) to classify square patch sequences in the processed images. The patches are categorized as either containing an exoplanet signature or only residual noise. The novelty of NA-SODINN lies in its ability to adapt to different noise regimes and incorporate additional features, such as signal-to-noise ratio curves, during training.
The study evaluated NA-SODINN against its predecessor SODINN, as well as other state-of-the-art detection algorithms, using ADI sequences obtained from the VLT/SPHERE and Keck/NIRC-2 instruments. The results showed that NA-SODINN outperformed its predecessor and other algorithms, especially in the speckle-dominated noise regime. NA-SODINN also achieved high rankings in the Exoplanet Imaging Data Challenge (EIDC), a competition for post-processing algorithms.
The findings of this research have important implications for the field of high-contrast imaging and exoplanet detection. By improving the accuracy of detection algorithms, astronomers can better identify and characterize exoplanets, leading to a deeper understanding of the demographics of directly imaged exoplanets. Although there are some limitations to the current NA-SODINN framework, such as its reliance on prior noise analyses and the challenge of setting an appropriate detection threshold, further developments and refinements are expected to enhance its effectiveness and practicality.
In summary, the NA-SODINN algorithm represents a significant advancement in the search for new exoplanets and offers a promising tool for analyzing individual datasets and large surveys using high-contrast imaging techniques. With its ability to work in different noise regimes and improve the detection performance, NA-SODINN holds the potential to contribute to groundbreaking discoveries in the field of exoplanet research.