Test-Time Distribution Normalization (TTDN) For Vision-Language Models
Published on Sat Oct 28 2023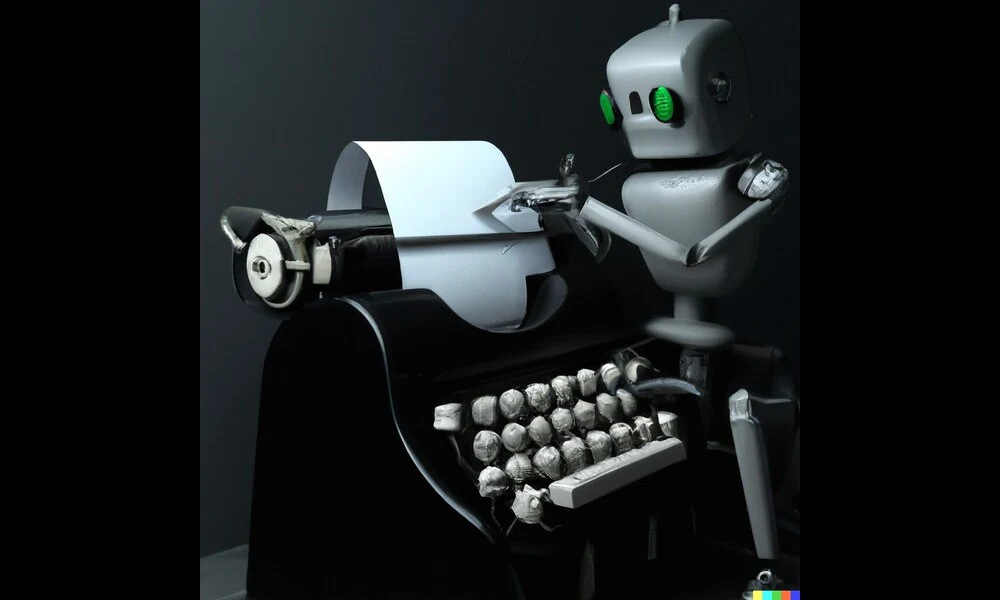
A team of researchers has made a groundbreaking advancement in the field of artificial intelligence by developing a novel technique called Test-Time Distribution Normalization (TTDN) for vision-language models. This new approach shows immense potential in significantly improving the performance and accuracy of these models, which are widely used in various applications, including language translation, image captioning, and more. The findings of this study, published in a preprint paper titled "Test-Time Distribution Normalization for Contrastively Learned Vision-language Models," are poised to revolutionize the AI landscape.
In this paper, the researchers focus on enhancing vision-language models, which are programs that can not only understand images but also read and generate text. Current models often struggle when it comes to accurately describing complex images or interpreting intricate textual prompts. They often make mistakes or generate vague, nonsensical responses. This is mainly due to the inconsistency between the training (learning) and testing (application) distribution of the models.
The TTDN technique proposed by the researchers aims to bridge this gap between the training and testing data distributions. By introducing normalization methods during the testing or inference stage, the model's performance can be significantly improved. TTDN ensures that the model adapts to the distribution of new data during testing, thereby generating more accurate and reliable results.
The implications of this research are far-reaching and exciting. Vision-language models, when enhanced by TTDN, become much more reliable and effective in performing tasks that involve analyzing and interpreting both images and text. For instance, this advancement can greatly improve automated image captioning systems, enabling them to generate more accurate and coherent descriptions of pictures. Furthermore, translation services can benefit from TTDN, as it helps language models translate sentences more accurately and consistently.
Importantly, this breakthrough has the potential to enhance communication between humans and machines. By improving the understanding of complex visual data and textual inputs, vision-language models can be integrated with virtual assistants and chatbots, offering more human-like and contextually appropriate responses.
The development of Test-Time Distribution Normalization is a significant step forward in the field of AI. The researchers' innovative technique enhances the performance of vision-language models by ensuring consistency during the testing phase. This breakthrough has the potential to revolutionize various applications, including image captioning, language translation, and human-machine interactions. As the integration of AI becomes increasingly prevalent in our lives, advancements like TTDN bring us closer to the dream of seamless communication between humans and intelligent machines.