Meta Researchers Create High-Precision Tree Canopy Maps
Published on Fri Jan 19 2024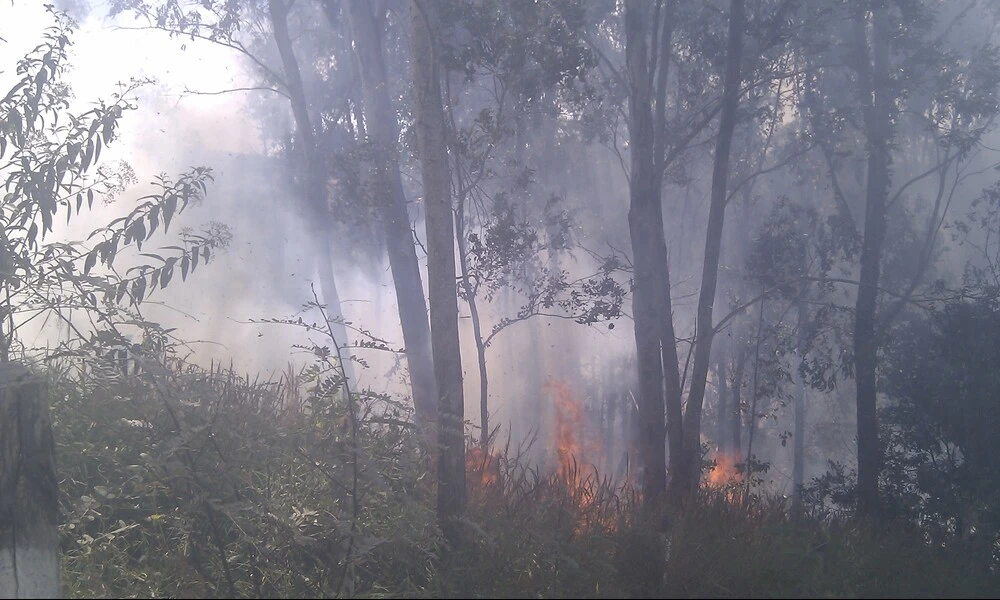
A new advance in environmental monitoring has been achieved by researchers from Meta's Fundamental AI Research (FAIR), the World Resources Institute, and the Desert Research Institute, who have developed a pioneering method to create extremely high-resolution maps of tree canopy heights. By utilizing advanced self-supervised learning techniques, these new maps offer unprecedented levels of detail, down to less than one-meter resolution. This is a substantial leap forward from the previous ten-meter resolution maps, providing a much more nuanced view of forest structures, critical for assessing carbon fluxes and understanding our planet's biodiversity.
At the heart of this advancement lies a self-supervised vision transformer paired with a convolutional decoder, which together have been trained on a combination of high-resolution satellite RGB imagery and detailed aerial LiDAR data. LiDAR, which stands for Light Detection and Ranging, measures distances by illuminating a target with a laser and analyzing the reflected light. However, until now, the scarcity of aerial LiDAR data has limited the production of comprehensive tree height maps. To overcome this limitation, the researchers trained their model using imagery from the states of California, USA, and São Paulo, Brazil, fine-tuned with observations from the Global Ecosystem Dynamics Investigation (GEDI) program.
The value of this research extends far beyond mere map-making. Vegetation structure mapping is crucial for a proper understanding of the global carbon cycle and for monitoring climate adaptation and mitigation efforts. For instance, measuring the height and projected area of tree canopies can help us observe phenomena such as deforestation, natural forest regeneration, and the implementation of sustainable agricultural practices, including agroforestry.
The researchers' findings, shared in their recent academic paper, detail a model that outputs canopy height with an average Mean Absolute Error of just 2.8 meters. In practical terms, this means the maps they produce could potentially help scientists accurately monitor carbon storage in forests at an individual tree level, something that was previously only possible through labor-intensive field measurements.
The maps generated from this research are already available and have been added to open data repositories, enabling further scientific study and potential application in environmental monitoring and planning. This represents a crucial step in using cutting-edge artificial intelligence to make sense of the wealth of data we can gather from our rapidly changing world. With this powerful new tool, researchers, policymakers, and conservationists will be better equipped to track and understand the Earth's complex forest ecosystems.