New Video-Language AI Model
Published on Mon Jan 15 2024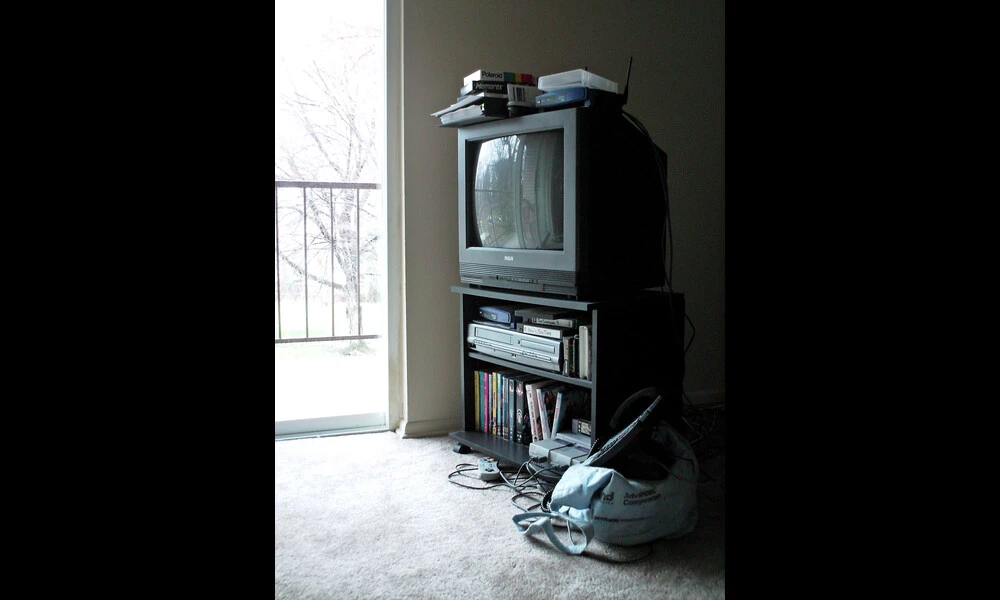
In the quest to enhance machine understanding of videos and their contents, researchers have made striking advancements using vision-language models, primarily leveraging vast amounts of image-text data. However, adapting these models to video data has been hindered by a shortage of large-scale human-annotated video-text pair datasets—until now. A team from Google and the University of Texas at Austin has developed a groundbreaking technique that enables image-language models to understand and generate high-quality captions for millions of videos. This newly adapted video-language model has not only demonstrated superior performance on a range of benchmarks but has also outshone previous state-of-the-art results.
Traditionally, creating video-text annotations has been a cumbersome process, often yielding limited and noisy datasets unsuitable for comprehensive training. To overcome this, the researchers fine-tuned an existing image-language model with synthesized video captions, leading to the generation of precise and informative auto-labels for a vast number of videos. This process was achieved through a sophisticated two-stage approach: first by adapting the video aspect of the model and then by fine-tuning the language component. The clever use of instructional data allowed the model to produce textual descriptions with varying levels of granularity—from basic appearance to specific body movements—maintaining the rich temporal information of videos.
The implications of this breakthrough are considerable. For instance, the enhanced model can outperform past benchmarks by sizable margins. On the NExT-QA benchmark, which involves open-ended video question answering, the new model exceeded the best prior result by 2.8%, showcasing its nuanced comprehension. Furthermore, the dual-encoder model, which leverages the automatically generated captions, showed a 3.8% improvement over the strongest baseline for contrastive training.
This advancement is not just a win for academia; it has everyday practicality. Imagine a search engine with the ability to accurately describe and index millions of videos, or assistive technologies that narrate video content for visually impaired users. These are just a couple of the wide-reaching applications that could arise from this innovative research.
In essence, this work exemplifies how smart adaptation of models, combined with synthetic data generation, can push the boundaries of what AI can achieve in understanding and interacting with the dynamic world of video content. It serves as a beacon for future explorations into the limitless potential of vision-language modeling.