Quality Metric for AI Conversations With Humans
Published on Thu Jan 11 2024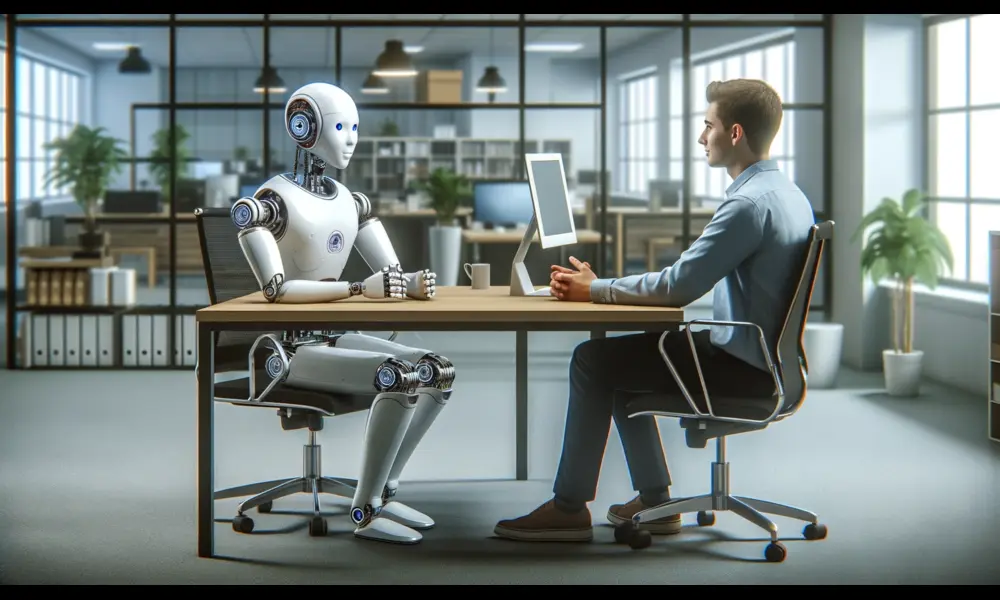
Having a conversation with a machine that can respond like a human is becoming increasingly mainstream with the growth in adoption of AI. But how does one measure how well these systems are doing? Sure, we could just ask people if they had a good experience — but in tech, we need something more concrete and comparable. Enter a team of researchers from Kyoto University and the KTH Royal Institute of Technology, who have developed a framework for objectively evaluating these spoken dialogue systems by examining users' behavior during conversations.
In their paper, soon to be presented at the International Workshop on Spoken Dialogue Systems Technology 2024, the team unravels how our spoken interactions, such as the number of words we use, our pauses in speech, and even our instances of "um" and "ah," can be telling signs of a system's effectiveness. The team discovered that in dialogues where the user's responses are key, like in job interviews or when simulating an attentive listener, features such as the number of user utterances and words are crucial indicators of a system's performance. In interactive conversations, factors relating to turn-taking, like how long it takes to switch from the system speaking to the user, are significant markers of system quality.
What does this mean for the average person? Essentially, by studying the naturalness of conversations with machines, researchers can tweak and improve systems to be more fluid and human-like. Instead of a one-size-fits-all approach, this study underscores that we must tailor our evaluation of these systems based on the specific type of conversation—what's important for a job interview bot might differ from a chatbot designed for more casual first-meeting conversations.
The researchers' analysis rests on a bedrock of rigorous data science methods, yet the implications are straightforward: the better we can understand and measure how humans interact with machines, the better we can make those machines serve us, whether in practice job interviews, customer service inquiries, or just having a chat. As the line between human and machine conversation continues to blur, studies like this ensure that our automated counterparts are keeping up with the complexities of human speech patterns. This isn't just academic—it's about improving the practical tools we use every day.