Study Reveals Gender Bias in ChatGPT Translations
Published on Wed Feb 14 2024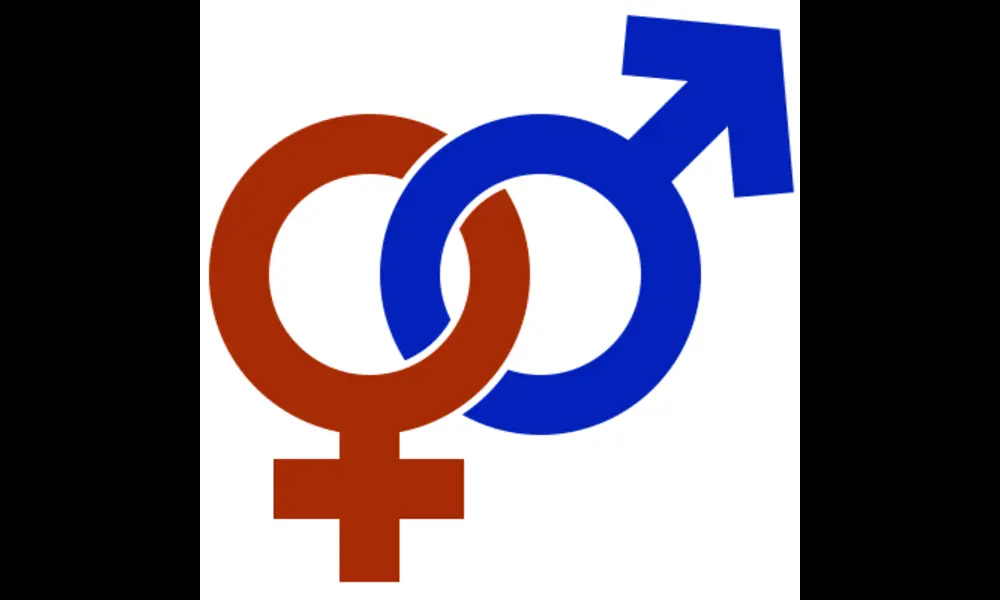
In an era where communication transcends borders at the click of a button, Machine Translation (MT) technologies like ChatGPT have become indispensable tools. Yet, in a recent study, Eva Vanmassenhove highlights a persistent shadow - gender bias in machine translation, particularly spotlighting the performance of ChatGPT in an English-Italian translation context. The findings, while expected, point towards the technology’s shortcomings in managing gender neutrality and inclusivity, underscoring a critical need for advancements in language technologies to foster fairness.
Vanmassenhove's preprint paper, titled "Gender Bias in Machine Translation and The Era of Large Language Models," offers a thorough examination of how MT systems, including the likes of GPT-3.5 based ChatGPT, can sometimes perpetuate or even amplify gender biases. The study, focused on cross-linguistic settings, delves into the heart of the issue - the inherent reliance of MT systems on statistical patterns which, coupled with dataset biases, can lead to problematic gender representations in translations. This revelation emphasizes the critical junction where technology and societal biases intersect, casting a spotlight on the ongoing challenges that language technologies face in mirroring and possibly exacerbating existing societal inequalities.
In an experimental setup, Vanmassenhove puts ChatGPT to test in translating gender-neutral English sentences to Italian. The results, to an extent, were startling but not entirely surprising. ChatGPT, despite its prowess, showcased a marked tendency to lean towards masculine translations, missing out on providing equivalent feminine or gender-neutral alternatives. This oversight reveals a significant gap in the model's ability to navigate the nuances of gender in translation systematically. Moreover, instances where the system was explicitly prompted to acknowledge gender, it surprisingly led to additional biases, highlighting a perplexing challenge in directing AI to mitigate embedded biases effectively.
These findings present a potent reminder of the monumental task at hand - ensuring that MT systems evolve to promote inclusive and unbiased translations. Vanmassenhove’s study brings to the fore the intricacies involved in debiasing language technologies and the multifaceted approach required to tackle such deep-rooted issues. It underscores the importance of a concerted effort across various disciplines, including computational linguistics, computer science, sociolinguistics, and ethics, to forge a path towards more equitable language technologies.
The implications of the study are far-reaching. As MT systems like ChatGPT continue to shape how we communicate across linguistic barriers, the urgency to address gender bias becomes paramount. The research not only sheds light on the critical need for ongoing advancements in language technologies but also champions the importance of fostering a collaborative environment that brings together diverse expertise to combat bias. The goal is clear - to harness the power of MT in breaking down not just linguistic barriers but also the barriers of bias and exclusion.
In essence, Vanmassenhove's research acts as a clarion call for increased accountability and innovation in the development and deployment of MT systems. It is an invitation to the global research community to prioritize fairness and inclusivity in the quest for evolving language technologies. As we tread forward, the hope is to see MT systems that not only translate languages but also transcend biases, embracing the rich tapestry of human diversity fully.