Forcasting Air Quality Impacts of Precribed Burns With AI
Published on Wed Dec 20 2023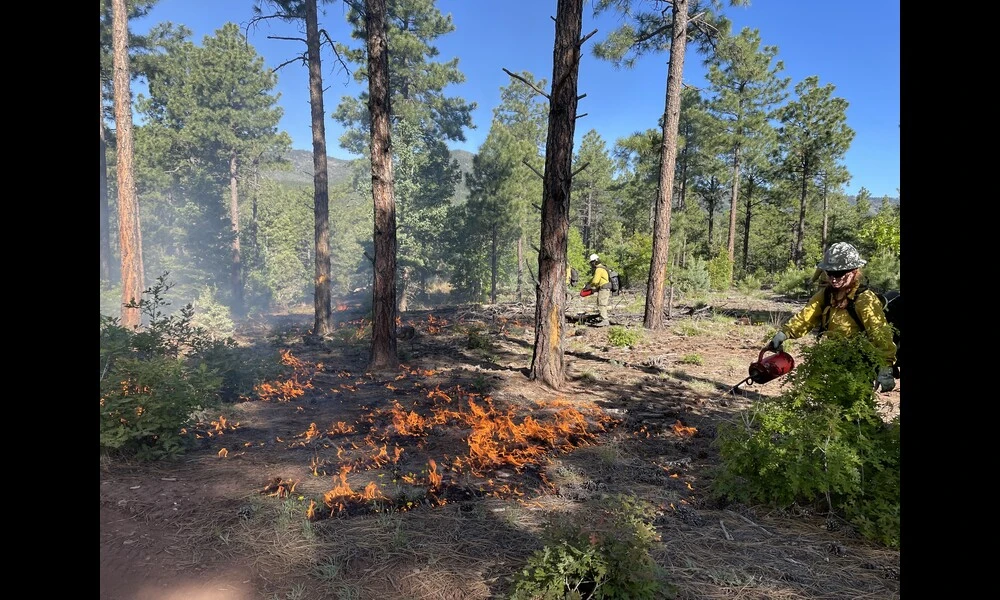
In a new study, researchers have harnessed the power of artificial intelligence to forecast the air quality effects of prescribed fires, offering a promising strategy to reduce the catastrophic smoke emissions from uncontrolled wildfires. The research presents a cutting-edge graph neural network (GNN)-based system that simulates the particulate matter emissions (PM2.5) from controlled burning — a key technique in fire prevention. This innovative tool could be instrumental for land managers striving to balance the benefits of prescribed fires with concerns about air pollution and public health.
Wildfires in western North America have not only intensified, but they've also turned the air smoggy with dangerous levels of PM2.5 — fine particles that pose serious health risks. The smart application of prescribed fires could help stem the smoky tide from future wildfires, but predicting their exact impact on air quality has been a tricky task. This preprint paper dives deep into the science of prescribed fires and the complexities of their planning, exploring how an advanced GNN can help chart the course for cleaner air quality amidst a changing climate.
The study's findings are particularly relevant for California, which has seen air quality improvements from the last two decades wiped out by recent extreme wildfires. By utilizing satellite observations and historical data on weather, fire behavior, and pollution, the researchers' model can predict PM2.5 levels, allowing for strategic scheduling of controlled burns that minimize negative effects on air quality. Put simply, this technical wizardry can find the perfect time for a prescribed fire that nips a potential wildfire in the bud — without leaving a blanket of harmful smoke in its wake.
The experiments cited in the paper paint a clear picture: not all times of year are equal when it comes to setting a controlled burn. The GNN-based forecasting system determined that the fall, particularly August, should be avoided due to already poor air conditions during peak wildfire season. Conversely, March presented a goldilocks window for these proactive burns—favorable due to comparatively lower PM2.5 levels, which suggests smarter, safer approaches to forest management are within reach.
In a nutshell, this novel integration of machine learning and environmental science doesn't just signal a leap forward in predicting smoke from prescribed fires — it also lights the way towards more informed decisions that protect both our forests and our lungs. As researchers look toward refining their model with even more sophisticated simulations, the study already stands as a beacon for those managing land and battling the ever-growing threat of wildfires.