Multilingual Social Media Tourism Analysis With AI
Published on Wed Dec 06 2023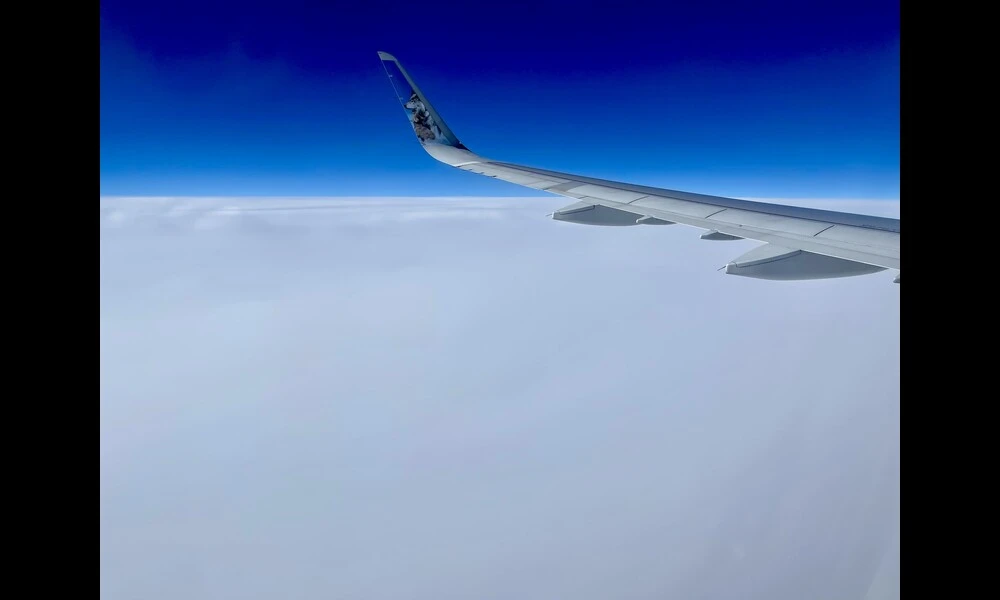
Imagine trying to grasp the ever-shifting trends in tourism by sifting through oceans of tweets and social posts—where every hashtag and emoji could hint at the latest hotspots or traveler grievances. This Herculean task, though, might just have gotten a whole lot easier, thanks to breakthroughs in machine learning. In a groundbreaking study published as a preprint, researchers have developed new strategies that can efficiently harvest insights from multilingual social content in the tourism sector with minimal human effort. Focusing on sentiment analysis, location detection, and thematic concept extraction, this research hinges on the use of large language models (LLMs) and a novel dataset, carving a path to a more nuances and real-time understanding of traveler sentiments and preferences.
The value of social media feedback in tourism cannot be overstated. It offers an unvarnished glimpse into what tourists care about and how they experience destinations. This new study tackles a key challenge head-on: the requirement for extensive and costly data labeling by humans. By pitting machine learning techniques against each other—namely few-shot learning, pattern-exploiting, and fine-tuning—the researchers discovered that competitive analysis in tourism can be achieved with astonishingly few annotated examples. Just 15 tweets were enough to gauge sentiment accurately, while approximately 160 were sufficient for location detection, and roughly 200 tweets allowed for detailed thematic categorization from a universe of 315 possible classes. This shift to leveraging large language models with scarce data marks a potential revolution for research and marketing practices in tourism, where being attuned to public sentiment is everything.
Importantly, this study doesn't just push technical boundaries—it also creates a bridge to practical application. The multilingual dataset itself—comprising English, French, and Spanish tweets related to tourism—is a unique resource that will be shared with the community, thereby providing a benchmark for future NLP endeavors in the tourism domain and beyond.
The implications of this research are far-reaching for tourism stakeholders and social science computationalists alike. With a reduced reliance on manual annotations and an escape from the confines of rule-based solutions, NLP practitioners now have at their disposal more streamlined, adaptable, and efficient tools to decipher the language of tourists globally. Whether for academics or for industry mavens, the insights gleaned from such analyses could prompt more agile responses to consumer trends, enhance destination marketing, and fine-tune services to match the latest traveler buzz—all while reducing the overheads of traditional data processing. As the researchers set their sights on creating dynamic dashboards to present their findings in an accessible format for industry partners, it is clear that this venture is not just an academic exercise; it is a step towards enabling smarter, data-driven decision-making in one of the world's most dynamic and influential sectors.