New Model to Improve Security of Autonomous Vehicles
Published on Sun Aug 13 2023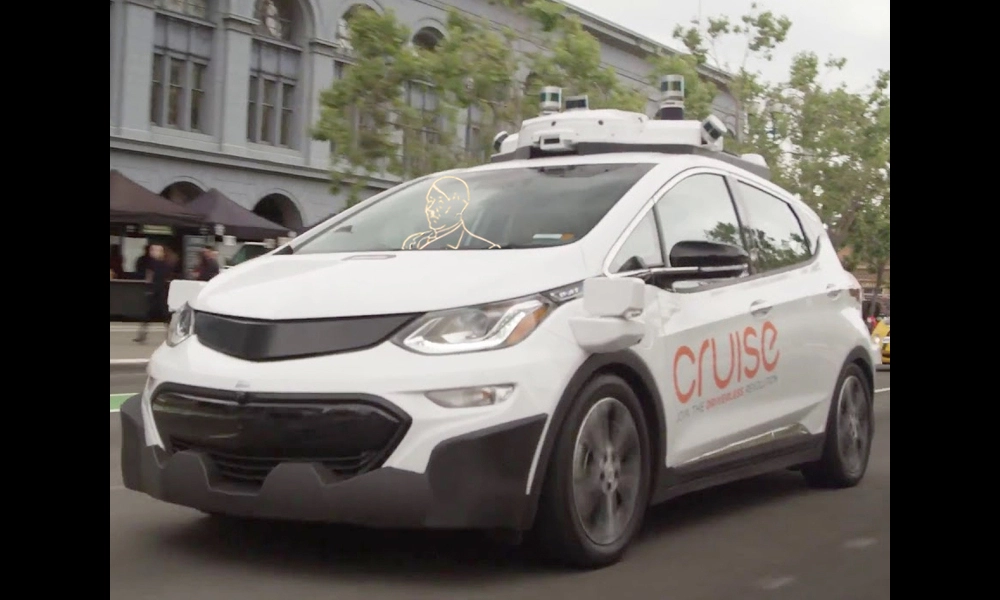
Autonomous vehicles (AVs) are becoming increasingly vulnerable to network attacks due to their high connectivity and diverse communication modes. In response to this, researchers have developed a new model called LSF-IDM, which aims to detect network attacks and provide functional safety and real-time communication guarantees for AVs. Unlike existing methods that struggle to detect concealed attacks within contextual features, LSF-IDM utilizes lightweight attribution and semantic fusion to effectively identify intrusions in in-vehicle networks (IVNs). This model combines a language framework called BERT with a lightweight model, such as BiLSTM, to capture semantic features and classify packet data. The results of experiments demonstrate the effectiveness of LSF-IDM in defending against various representative attacks.
With the rise of modern vehicles equipped with electronic control units (ECUs) that manage vehicle functions through IVNs like the Controller Area Network (CAN), there has been an increasing concern for security vulnerabilities. IVNs, which are open to external networks, have weaknesses in access control, confirmation, and encryption, making them susceptible to cyber-attacks. Malicious hackers can insert precisely designed packets into the CAN bus to compromise the pre-defined protocol of the vehicle. This can result in attackers gaining control over critical subsystems like the engine, steering wheel, and braking system, posing not only a security risk but also a functional safety concern. To protect against such attacks, measures like encryption, authentication technologies, firewalls, and Intrusion Detection Systems (IDSs) are used. However, IDSs face limitations in terms of limited computing and storage resources, numerous attack interfaces, and high real-time and reliability requirements.
Traditionally, IDSs relied on methods such as specification-based, statistics-based, and physical fingerprint-based techniques to identify intrusion messages, but these methods often perform inadequately. Recently, there has been a shift towards using deep learning (DL) methods that model traffic behavior to detect abnormality. However, these DL-based IDSs struggle to extract valuable features using increasingly complex model structures. Some research has focused on using pre-trained language models like BERT and GPT-2 to analyze the context feature of transmitted messages. Although these strategies have shown promising results, implementing them in resource-limited environments like IVNs is ineffective. Another challenge is the high false alarm rate and performance bottleneck in lightweight models.
To overcome these challenges, the LSF-IDM model utilizes a pre-trained language model as a teacher model to extract complex contextual semantic features. These valuable features are transferred to a lightweight model through knowledge distillation. This fusion of features enables LSF-IDM to detect abnormal traffic more effectively, addressing the performance bottleneck arising from balancing detection performance and model complexity. The experimental results on a benchmark car hacking dataset demonstrate the superiority of LSF-IDM in terms of detection accuracy and false alarm rate compared to traditional machine learning methods. Importantly, LSF-IDM provides a practical solution for resource-limited environments like IVNs.
The LSF-IDM model presents a significant step forward in enhancing the security of autonomous vehicles by effectively detecting network attacks. By combining a pre-trained language model and a lightweight model through knowledge distillation, LSF-IDM achieves improved detection accuracy while addressing the limitations of computational resources and performance bottlenecks. Future research can explore the implementation of LSF-IDM with different pre-trained language models and further investigate knowledge transfer techniques. The development of such advanced security measures is crucial as autonomous vehicles continue to advance and integrate more extensively into our daily lives.