Improved Video Classification Leveraging Audio Data
Published on Sat Sep 09 2023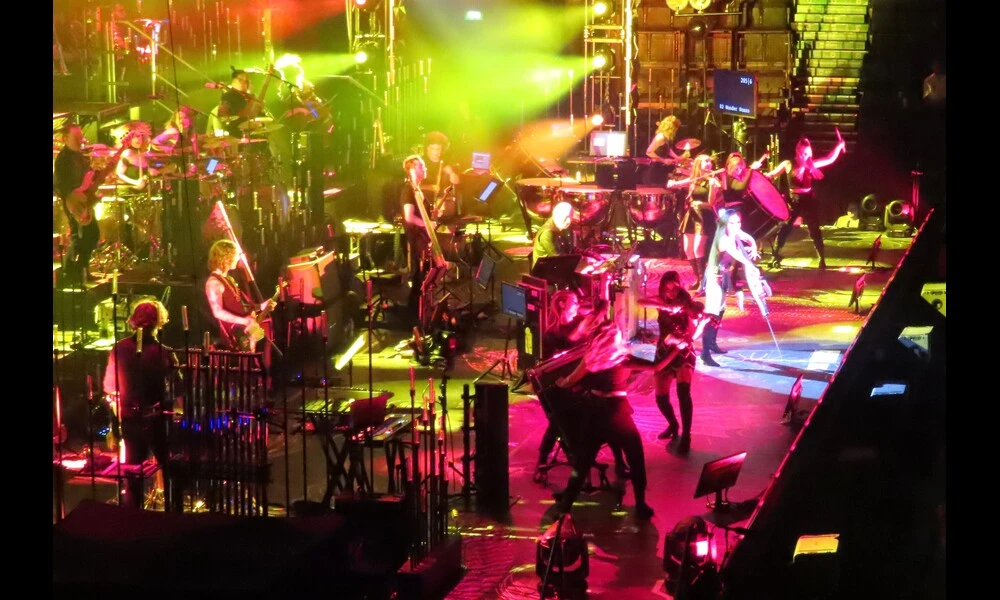
A new research paper introduces a groundbreaking method for few-shot learning in video classification using audio-visual data. Traditional video classification models require vast amounts of labeled training data, which can be expensive and time-consuming to gather. However, this study explores the potential of few-shot learning, which enables models to recognize new classes with only a few labeled examples. By leveraging the multi-modal nature of video data, which combines visual and sound information, the researchers introduce a unified audio-visual few-shot video classification benchmark on three datasets. They propose a text-to-feature diffusion framework called AV-Diff, which combines temporal, audio, and visual features using cross-modal attention. This novel approach achieves state-of-the-art performance on the benchmark, showcasing its effectiveness in audio-visual (generalized) few-shot learning.
The use of audio-visual data in video classification has shown promising results due to the complementary knowledge found in both modalities. However, traditional video classification models rely heavily on vast amounts of expensive training data. To address this issue, the researchers focus on few-shot learning, which allows models to recognize new classes with just a few labeled examples. This approach not only reduces the need for large-scale labeled data but also leverages pre-trained visual and sound classification networks to operate on the feature level, making it more computationally efficient.
In this study, the researchers specifically tackle the task of few-shot action recognition in videos using audio and visual data. They focus on the generalizes few-shot learning (GFSL) setting, which requires the model to recognize samples from both base classes (with many training samples) and novel classes (with only a few examples). The researchers emphasize the importance of additional modalities, such as text and audio, for learning robust representations from limited samples.
To evaluate their method, the researchers introduce a new benchmark for audio-visual GFSL for video classification. This benchmark consists of three audio-visual datasets and includes ten carefully adapted methods for comparison. The proposed model, AV-Diff, uses a hybrid attention mechanism to fuse audio-visual information and a text-conditioned diffusion model for generating features for novel classes. By effectively combining these components, AV-Diff achieves superior performance compared to other state-of-the-art methods on the benchmark datasets.
Overall, this research paper opens the door to more effective audio-visual classification when only limited labeled data is available. By leveraging the multi-modal nature of video data and incorporating a text-to-feature diffusion framework, the authors demonstrate how few-shot learning can be a powerful tool for efficient video classification. This advancement has the potential to benefit various fields, such as computer vision, where accurate and real-time video classification is crucial.