New Metric Improves Cardiac MRI Analysis
Published on Wed Aug 30 2023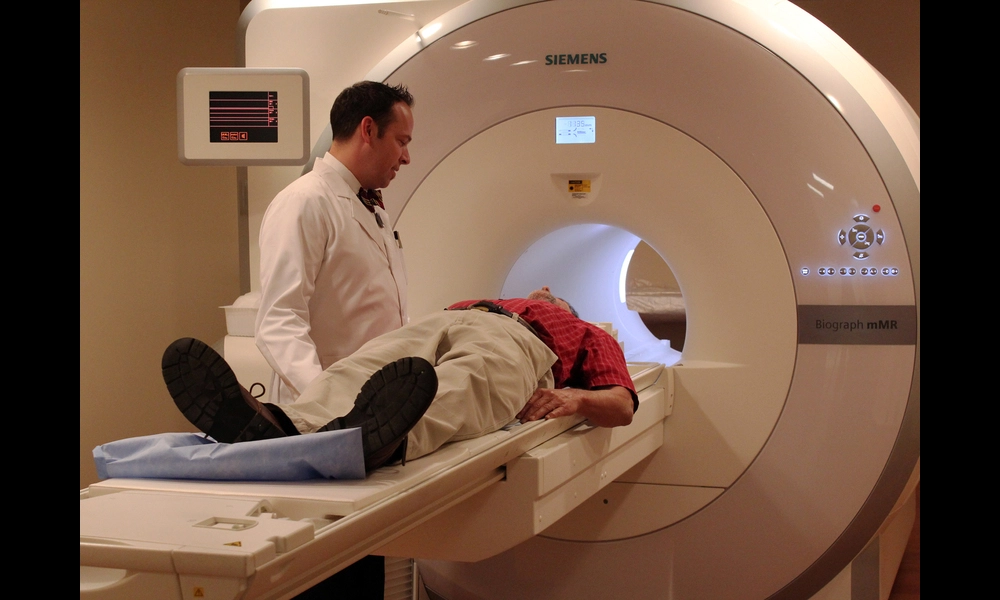
Dynamic contrast-enhanced (DCE) cardiac magnetic resonance imaging (CMRI) is a crucial tool for diagnosing heart conditions. However, the manual segmentation of DCE-CMRI datasets can be time-consuming and challenging. To address this, researchers have developed a dynamic quality control (dQC) tool that uses deep neural networks (DNNs) to detect and improve failed segmentations. In this study, the researchers propose a new space-time uncertainty metric as a dQC tool for DNN-based segmentation of free-breathing DCE-CMRI datasets, allowing for more accurate and efficient analysis of the images.
The proposed dQC tool identifies the top 10% most uncertain segmentations and refers them to a human expert for refinement. By using this approach, the researchers achieved a significant increase in the accuracy of the segmentation results and a notable decrease in the number of images with failed segmentation. In contrast, randomly selecting the same number of segmentations for human referral did not yield any significant improvement. These findings suggest that the dQC framework can accurately identify poor-quality segmentations and enable more efficient and reliable analysis of DCE-CMRI datasets.
The proposed dQC method leverages patch-based analysis to assess the quality of DNN-derived segmentations for each DCE time frame. The results demonstrated that when only 10% of the dataset was referred to a human expert for correction, the dQC-guided selection significantly improved the performance of the segmentation compared to random selection. Moreover, the dQC-guided corrections led to a lower prevalence of failed segmentations, which is crucial for accurate quantitative analysis of DCE-CMRI data.
While there is subjectivity involved in grading the difficulty of segmentations, the researchers incorporated clinical expert feedback to mitigate this limitation. This study represents an important step towards improving the analysis of DCE-CMRI datasets through an innovative dQC tool. Future research can explore further refinements to the dQC metric and expand its application to other medical imaging modalities. With enhanced segmentation accuracy and efficiency, clinicians can rely on DNN-based analysis in a human-in-the-loop pipeline for more precise clinical interpretation and reporting of dynamic CMRI datasets. Overall, this promises improved diagnostic capabilities and better patient care in the field of cardiac imaging.
New Metric Enhances Accuracy and Efficiency in Cardiac MRI Analysis