TacoGFN: A New Tool for Structure-Based Drug Design
Published on Thu Oct 12 2023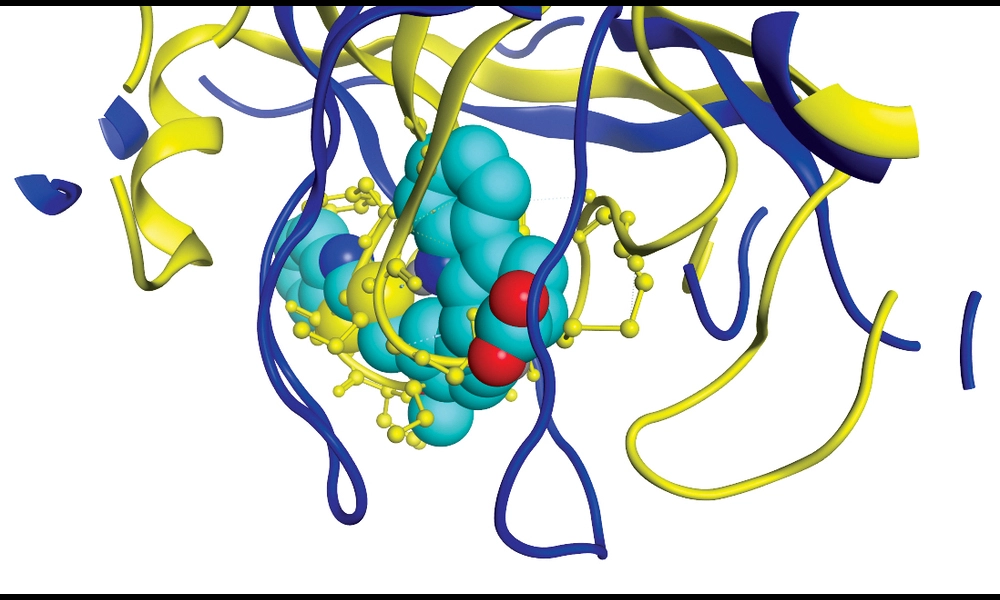
Researchers have developed a new method called TacoGFN that aims to automate the generation of drug-like compounds for specific protein pockets, a process known as structure-based drug design. Current methods struggle to generate molecules with significant binding improvement over the training dataset, limiting their effectiveness in drug discovery. TacoGFN, on the other hand, uses a reinforcement learning approach to generate molecules with desired properties, such as high docking scores and drug-likeliness. The method incorporates a docking score prediction model and active learning to improve the generalization of the generated molecules. Empirical results show that molecules generated using TacoGFN outperform all baseline methods in terms of docking scores, synthetic accessibility, and drug-likeness, while being much faster.
Structure-based drug design is an important approach in drug discovery, leveraging protein structures to design and optimize potential drug molecules. However, traditional methods like molecular docking have limitations in searching virtual libraries of molecules for interaction with a target protein. To address this, generative models for molecules have been proposed, which turn the virtual screening problem into a search problem. TacoGFN is a novel method that takes this approach further by learning a policy to generate molecules with probabilities proportional to their reward, based on docking scores and desired properties. This approach allows TacoGFN to explore a much larger chemical space compared to traditional virtual libraries.
One key advantage of TacoGFN is that it does not rely on a fixed dataset to generate molecules. Instead, the method focuses on exploring the chemical space and generating molecules with better properties than the reference dataset. This is achieved by using the GFlowNet model, which constructs objects with probabilities proportional to their reward, ensuring a diverse set of solutions. The researchers also incorporated an active learning approach to gradually improve the generalization of the docking score prediction model, which further improves the quality of the generated molecules.
Experimental evaluations on unseen pockets showed that TacoGFN consistently generates molecules with better docking scores and properties compared to existing methods. The method outperforms all baseline methods in terms of molecular properties, including the widely used Vina Score. In addition, TacoGFN is significantly faster, generating molecules 50 to 1000 times faster compared to other methods. This makes TacoGFN a valuable tool for structure-based drug design campaigns.
Overall, TacoGFN represents a paradigm shift in generative approaches for structure-based drug design. By focusing on exploring the chemical space and generating molecules with desired properties, TacoGFN outperforms existing methods and offers great value for real-world drug discovery campaigns. Further research could explore leveraging uncertainty estimation, improving pocket representation, and incorporating known reactions for molecule generation.